Rebooting Materials Discovery and Design
- By Michael Haverty
- •
- 22 Jan, 2018
- •
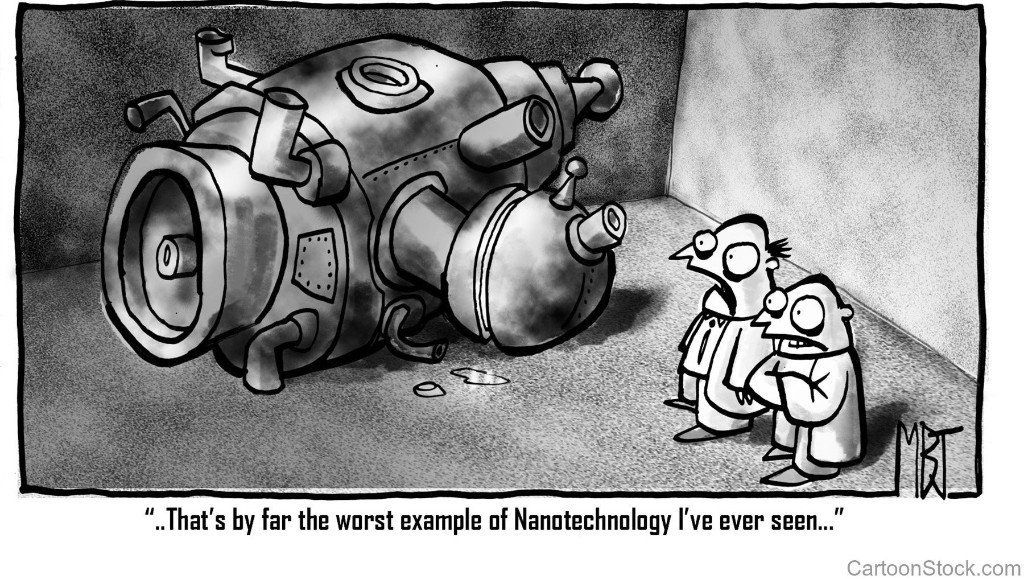
I mentioned in my previous blog my challenge to the common question I am asked about the "next steps for materials discovery.” I want to dive into more depth as this is central to the focus of my blog and even motivated the blog name Rebooting Materials. In my current role I speak with a wide variety of companies from start-ups to Fortune 500, from people at universities to National Labs. I always stress the real challenges that exist in taking good ideas in research and development (R&D) of new materials and chemistries and translating them into impacts on a real world product especially at the nanotechnology scale.
I am primarily a theoretician in training who focuses on applying models to generate new insight and ideas. When I started my career, it was easier to work and think mostly in the world of the models I used. If I hadn’t learned later in my career to keep my feet firmly planted in the real world, I wouldn’t be where I am today, but that doesn’t mean there weren’t bumps and setbacks along the way. To turn in a bit of a philosophical direction though, I think a lot of scientists fall into a similar trap and become a bit too wedded to their ideas and training.
Mistaking models for reality:
To explain, I’d like to take a slight diversion into the general world of physics and talk about the example of wave-particle duality of a photon and an electron. It's over-simplification, but one can say that both the photons we see as the sunlight delivering light and warmth into our lives and the electrons around the nuclei of all atoms and elements in our body sometimes act like a wave on an ocean and sometimes act like a billiard ball on a pool table. The words in that run-on sentence that I want you to focus on and think deeply about sentence are “act like.” The crux of my point is that a photon or an electron are not a ball or a wave but something else entirely that shares some properties of both (if you want to dive deeper here, check out the double-slit experiment.)
The word “ball” is an approximation and an idealized way to characterize reality. It’s very useful to explain how a variety of different things (an orange, a collection of twine, a baseball, or the earth) all share similar characteristics. If you think about the underlying structure, those 4 “balls” all are vastly different even though one could accurately refer to them all as a “ball” at some level. (Ok, maybe the baseball really is the closest to being a true “ball”, but let's not go down the rabbit hole of why are a basketball, soccer ball, and football are all “balls” as well. I’d like to keep this blog shorter than a thesis after all!)
Back to the real world after that diversion:
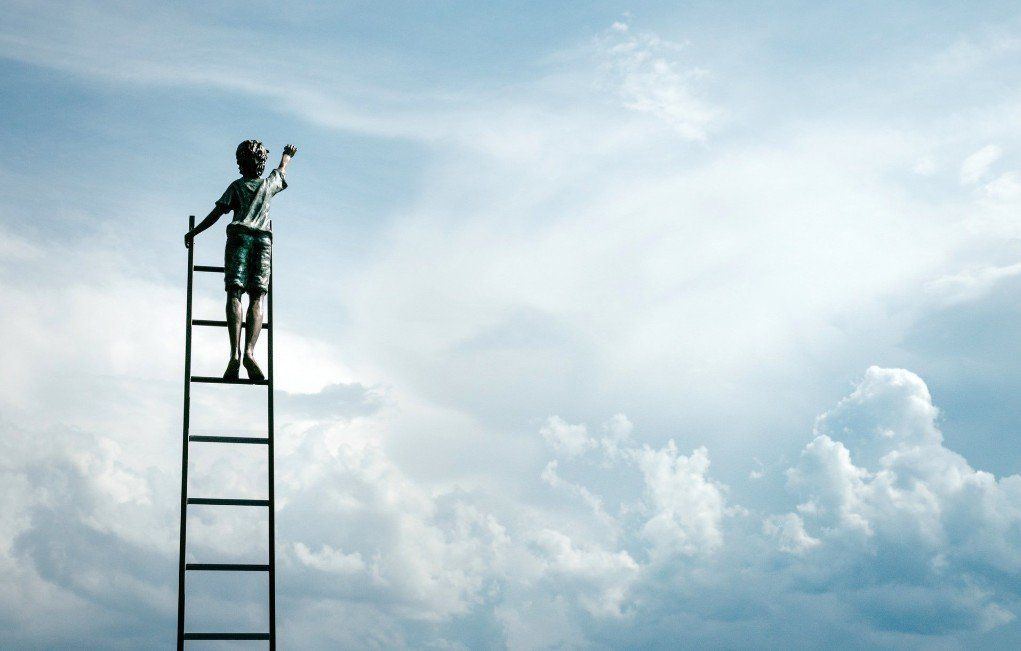
Early on in my career I fell into the trap of forever trying to prove my models and theory were correct. Comparison with data was always the most important thing and the struggle to get a prediction error somewhere less than 5 or 10% was the goal. That’s a laudable goal, but to be frank the real world of R&D is very messy has too many unknown variables. Even if you perfectly predict an idealized property of a homogeneous material, its value is limited in a complex system of defects when multiple materials are in contact with each other at nanoscale dimensions.
Remember those bumps in the road along the way? Here’s an example:
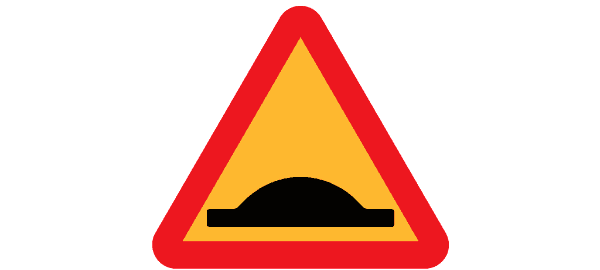
In the past I worked with experimentalists screening metallic materials for high electrical conductivity. As a modeler, I’m used to walking into a room of questions (What value I can provide? Can I be trusted? Will I waste other people’s time?) This interaction had that healthy dose of skepticism and then some. We decided to start with a detailed validation study comparing our model with their experiments on a material-by-material basis. For one material we struggled to predict with less than a 20% error. This formed a real stumbling block to a productive collaboration.
We went into deep-dive mode to examine what might be wrong. We asked the experimentalists every question we could think of about the material (deposition, microstructure, thickness, roughness, variation, etc.) After more than a month of unproductive back-and-forth rerunning our models, double and triple-checking our assumptions, we reached an impasse.
At this point I had a casual hallway conversation with the researcher about the material. He nonchalantly mentioned the deposition technique may leave ~15% of non-metallic elements in the final material. This hit me like a wrecking ball. The most critical input into our model was to make the atomic structure and composition most closely reflect reality as possible. I actually stopped him a number of times just to confirm and re-ask the same questions while trying to hide my annoyance. In the process I also probably ended up annoying him as well.I couldn’t believe what he had just said. Everything I had simulated for that material up until that point had been wrong up until that point. With that critical piece of data updated we were able to construct the right structure and the prediction matched the experimental data.
Who do you think was at fault in the above story?
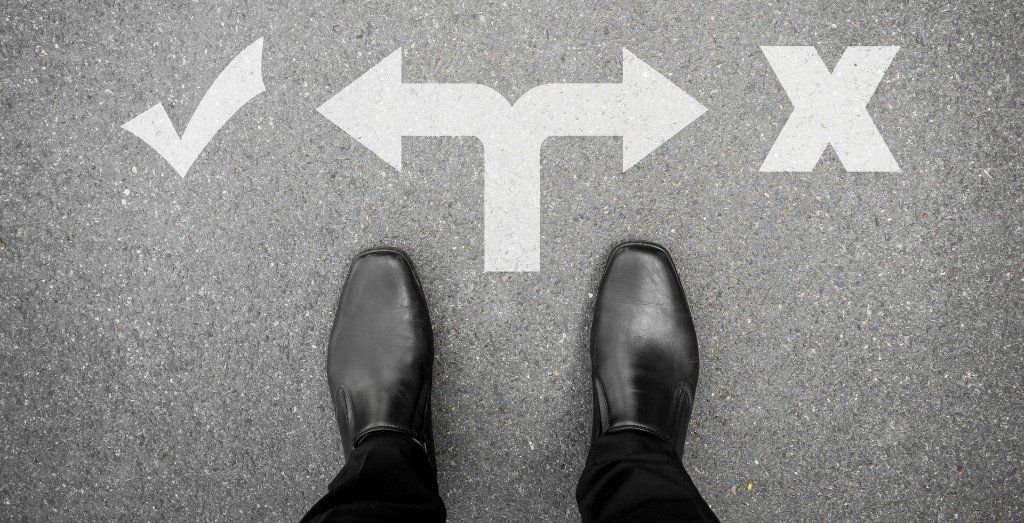
If you’re a modeler, you’d probably say the experimentalist was a jerk and should have thought to tell you that critical detail. If you’re an experimentalist, you might say the modeler sucked at communicating and never really told you what you needed to know or asked that question. Today, although I must admit my opinion evolved over time 😊, I think we were both at fault.
Our collaboration started off on the wrong foot with a distrust that prevented effective discussion of all the information that we both needed to help each other. I needed to know some of the trouble he had to deal with in making his material. He needed to understand some of the complexities of my model to appreciate the critical pieces of information I needed. Due to starting off on the wrong foot we didn’t get to the more important task of pushing the needle on the speed of R&D until too late in the game.
Bringing it back to Materials Discovery and Design:
With the advantage of hindsight and many more years of experience I see this as a pretty typical interaction between modelers and people generating data in the real-world. All too often when a project starts, the modeler dives into proving their technique for predicting a property. Once proven to the satisfaction of the experimental partner the model turns into a crank to turn out results and recommendations (i.e.: discovery). The experimentalist becomes a disinterested party just watching and waiting to see what the modeler can produce and tends to look for holes to poke in the results. This tension between supposed partners is often reinforced by the fact that under this scenario it may appear that the modeler is trying to replace the experimentalist.
I worry about those that are focused only on high-throughput prediction and study to identify the next greatest material for battery, catalyst, dielectric, or 2D material applications. Discovery is an important step in the process and data-centric techniques have an role to play in the process. However, without a deep understanding of the eventual target manufacturing process and the design of how that material will integrate into a final product only focusing on discovery threatens to just produce a large volume of data that amounts to nothing. In some cases the discovery may even be valid, but when tested experimentally the signal is hidden in the noise or some other complication and then forgotten or ignored.
How much discovery and how much design?
I was giving a talk in Lawrence Livermore recently and got into a discussion about how critical it was for the modeler to think about the design and integration parts of a project. After some introspection (Thanks for the thought-provoking question Vince!) I believe a good way to think about this is to estimate the number of processing steps, the number of different materials, and the number of layers that are used in producing your finished project.
If you have a product like an integrated circuit (IC) from the semiconductor industry the need to think about design and integration is an absolute. Many semiconductor chips use ~10’s of different processing techniques on 10’s of different materials many of which are used repetitively in building ~10 different functional layers. (Think of the “layers” in a integrated circuit as floors in a building built of multiple materials. Check out semiconductor device fabrication for a deeper dive.) Even if you come up with a ground-breaking new material to replace one of those 10 materials it’s still probably going to touch 2 to 4 of the incumbent materials and be exposed to 2 to 4 of the processing techniques in 2 to 7 of those layers.
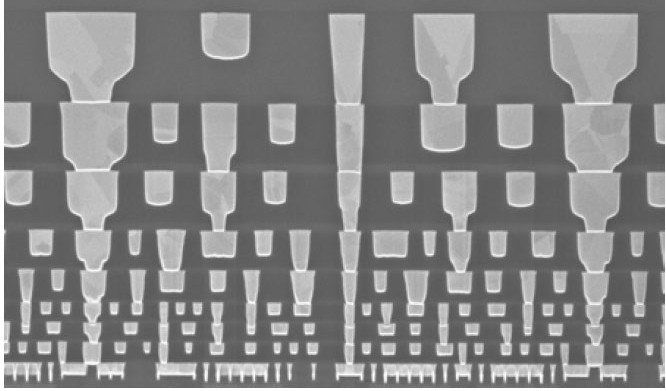
That’s a LOT
of chances for error and unexpected things to
happen. Discovery alone simply doesn’t
even scratch the surface of all that is possible in this regime. You need to understand the experimental
production and testing processes well-enough to suggest meaningful and
insightful designs of experiments
(DOE's) to gather information that is too
costly or complex to simulate. You need
to think about the design of how your new material or chemistry will interact
with everything that you can tractably model with your technique.
This also has implications on the level of detail and accuracy you can tolerate in your model software to enable you to examine all of these effects. I think this perspective calls for a bit more pragmatism than has been implemented in the materials and chemistry modeling communities up until now, but that’s a topic for another future blog.
Less complex industries still need design of materials for product integration:
There are other industries with great materials’ challenges that don’t have the degree of integration and complexity of integrated circuits. Large-scale structural alloys and steels come to mind as they are used in largely monolithic components, although they do include a wide variety of elements in their formulation. Solar cells and batteries require far fewer layers than a semiconductor chip so the design and integration requirements are lessened there as well. Even for these larger scale technologies however, you still have multiple materials coming together into contact and being processed with different methods to chemically or mechanically bond together. To focus only on discovery ignores the complications that arise at these steps. The challenges for modeling in the catalyst industry are less about the interaction with other materials and more about the design of the model test plan to sample the all relevant chemical pathways and steps that may be accessible.
One might argue that all these integration issues might be devolved into a metric(s) to be included in the discovery methodology and brute force of high throughput computations, or turn to another database that could be used to shake a certain way to get out the right answer. In practice, I’ve found these integration issues tend to require another order of magnitude of computing power to estimate due to their complexity or time scale. For data-centric approaches I’m concerned the density of science and engineering data available isn’t sufficient for sampling options outside the limited scope of what’s already been explored.
A proposed high-level design methodology:
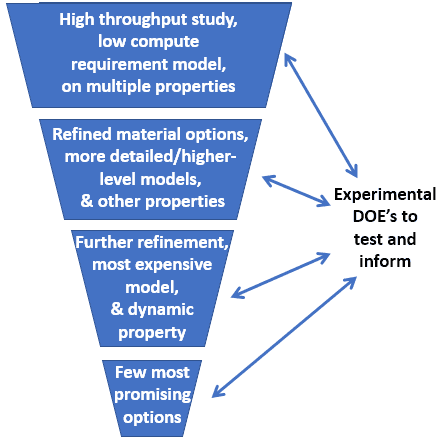
I argue that what is needed is to design your models in a funnel type of approach where the matrix of options is slowly down-selected by varying levels of theory and software in close collaboration with experimental DOE’s as sanity checks and sources of additional information along the way. That isn’t too say that I don’t think there should be multiple criteria embedded at each level of theory. The name of my effort Property Vectors comes from this concept that experts need to make sure they estimate multiple properties (or a “vector” of properties). Said another way with a current buzzword, you might call this idea co-optimization of multiple properties. Either way, this effort needs to be executed on multiple material options when trying to decide on what atomic structures to move onto the next level in the funnel in the design methodology. In practice I prefer for the final recommendation to be a set of materials. No matter how many complications you tried to test for in your modeling and experimental pre-work, surprises will always come up later so it’s best to hedge your bets. 😉
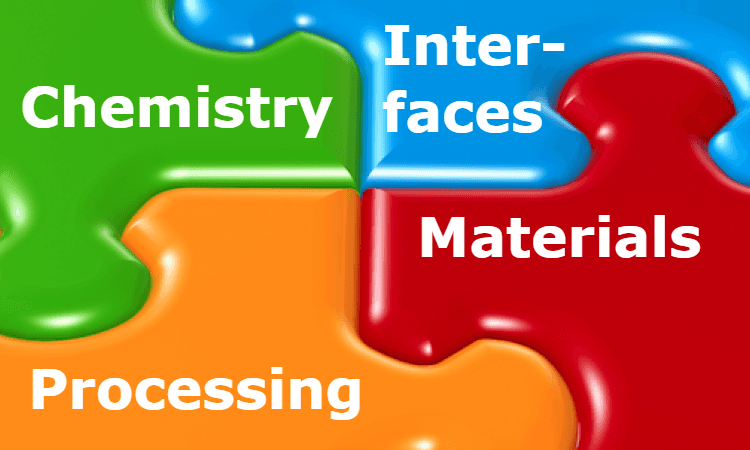
What’s in a name?
Wrapping this all together is why I named my blog Rebooting Materials. There have been a few false starts in the demonstrated and sustained use of atomic-scale modeling techniques to make wide impacts to industrial R&D. Part of this has to do with the software tools, but part of it also has to do with the practice by people (modelers and experimentalists). I think both aspects need a “reboot”.
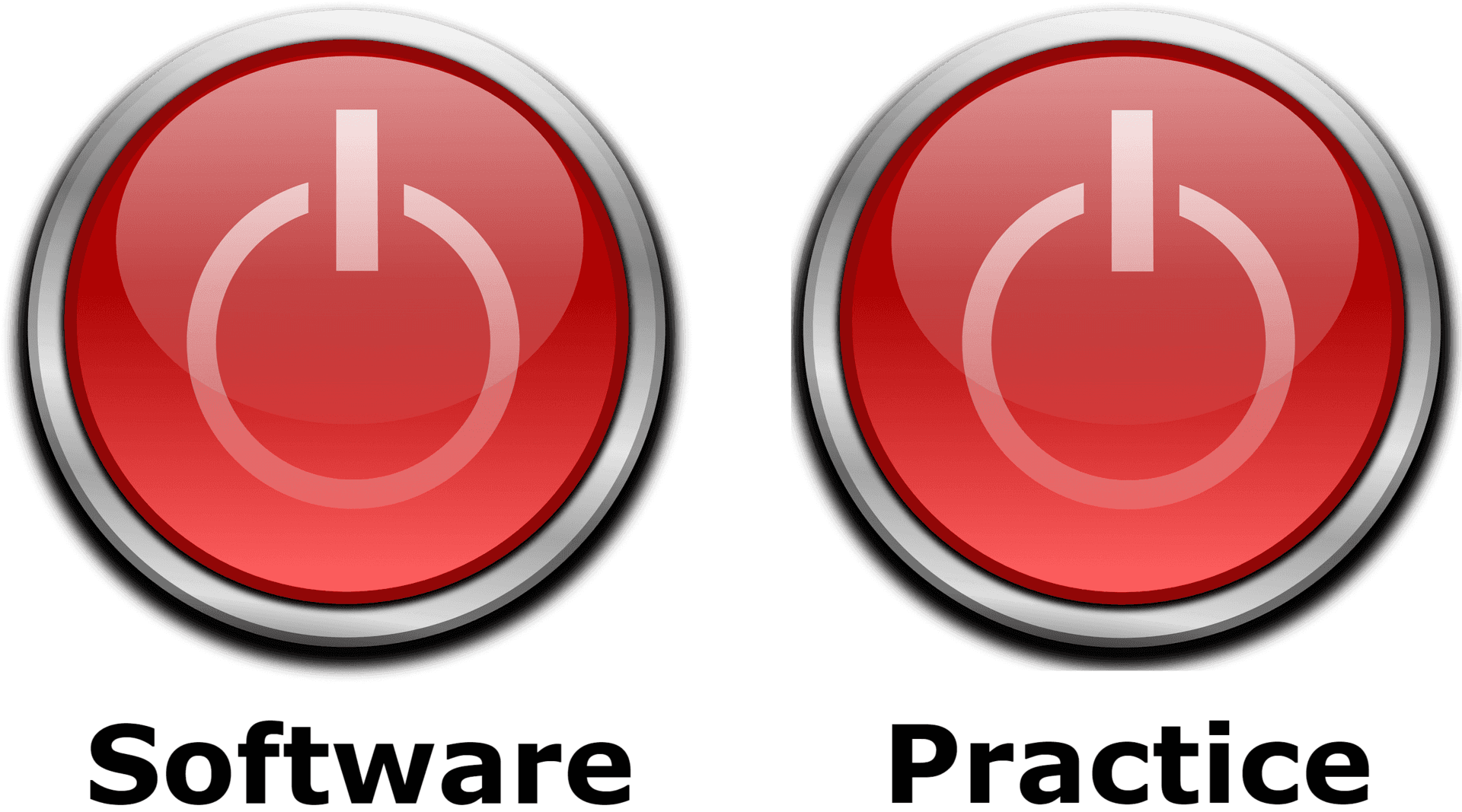
Modelers need to stop trying to replace experimentalists and
start looking at them as their best source of information to fill in their
holes. They need to embrace multi-scale
efforts in all their complexity and difficulty and do their best effort to
bring the gaps between various levels of tools.
Experimentalists need to play a role as well and embrace modelers more
to rapidly test out their own theories and not reflexively shut the door on
them if a prediction gives more than X% error.
Software tools and their developers also need to be more pragmatic. Think of tools like Ansys, AutoCAD, Solidworks, and even Synopsys. Those tools don’t promise 100% accuracy. They offer a friendly interface to powerful engineering software and ease the construction of modeling workflows. These tools take some of the decision making away from the user to shorten the learning curve and speed the path to productivity. Schrodinger is a good example of an atomic scale tool that simplifies the use of difficult techniques, doesn’t promise 100% accuracy, but successfully delivers dependable capabilities to a wide cross-section of companies across the drug discovery and design industry.
We need to get closer to a state where building the atomic structure of a new material and estimating its properties in software is as easy as designing a new airliner and as critical to the early stage R&D process as the finite element tools of today are to manufacturing design and development stages. I submit these high level ideas as a first step of my suggestion of how to get further down this path and would love to hear your thoughts as well!
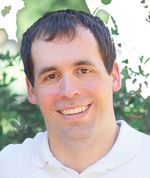
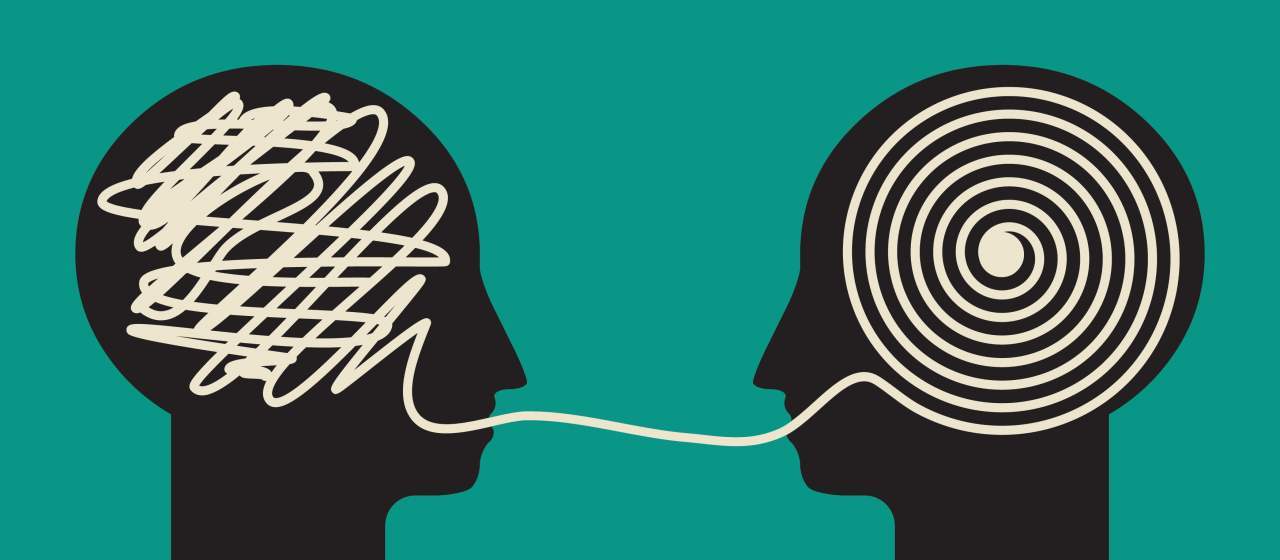
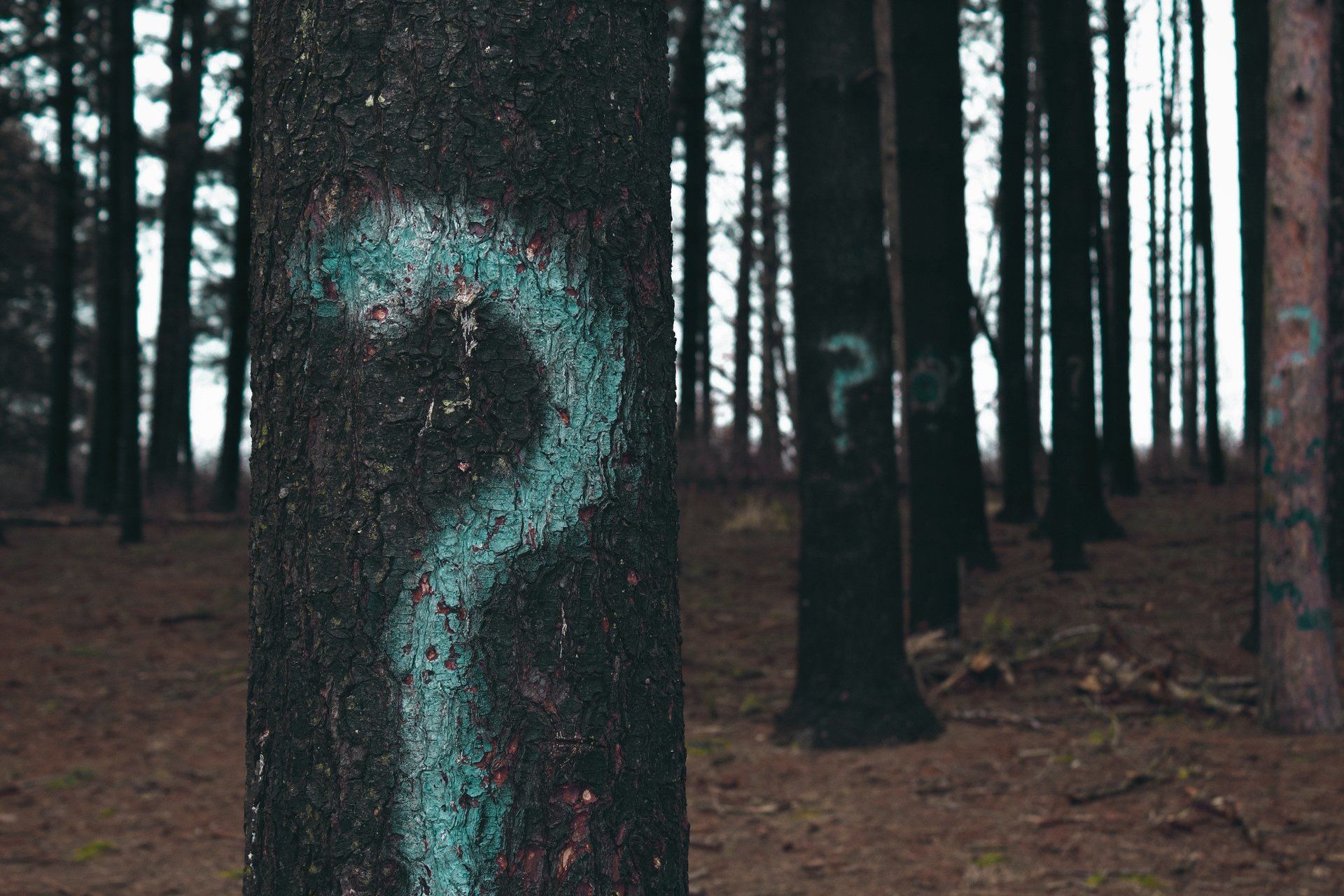